Beef Cattle Selection Using Data Analytics to Increase Genetic Gain
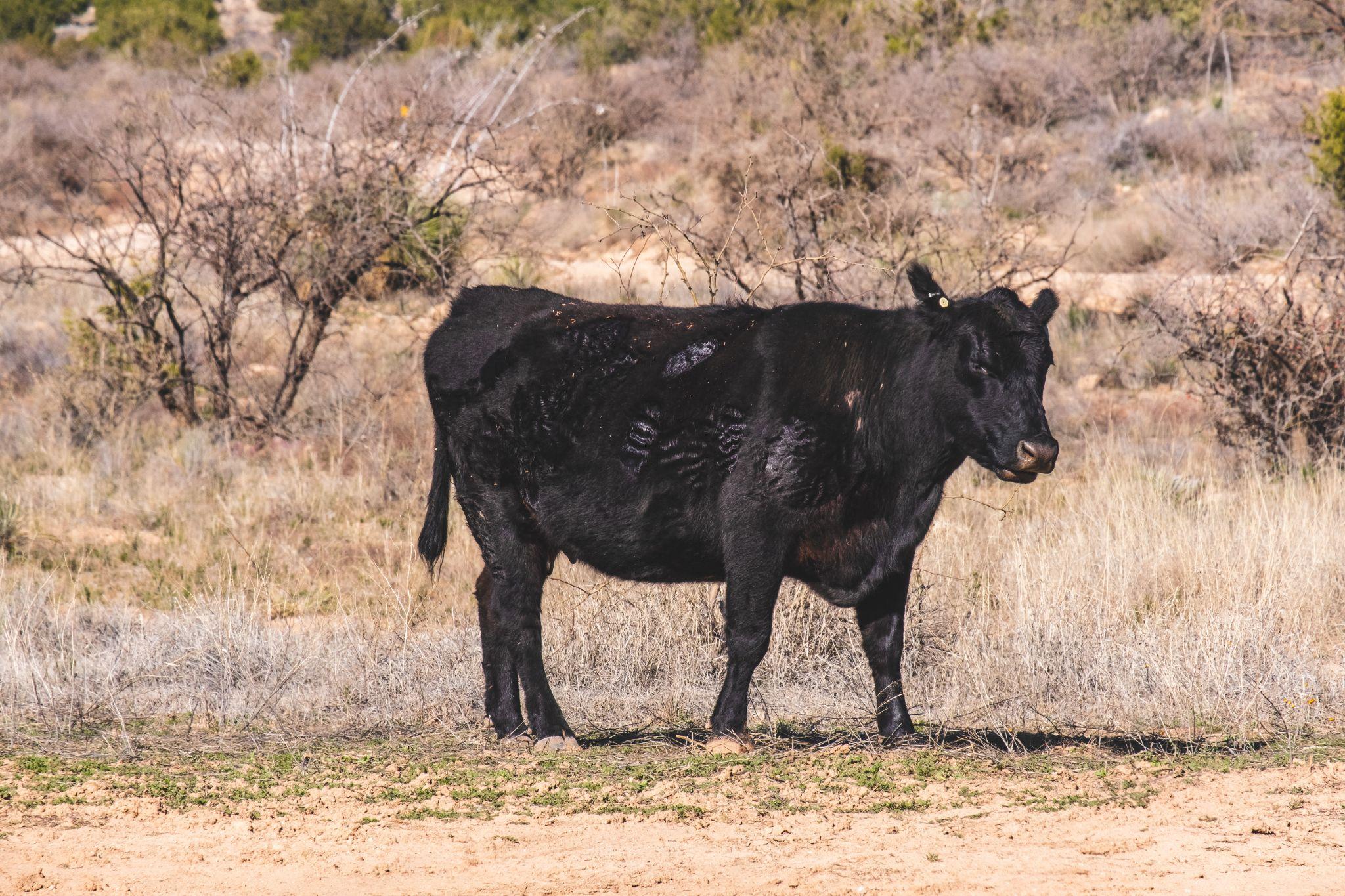
Cow in a grass field
Genetic gain in cattle can be defined as the amount of performance improvement achieved annually through selection, and cattle breeders are attempting to improve genetic gain year after year as demand increases. Factors affecting genetic gain include genetic variation, heritability for traits of interest, selection intensity, and the time required to complete a breeding cycle. Modern breeding techniques which use data analytics offer new opportunities to enhance genetic gain, shorten the breeding cycles and improve the selection intensity.
Let us explore how data analytics can help increase genetic gain in beef cattle.
How Data Analytics Improves Beef Cattle Selection?
With recent developments in data recording and analytics tools, it is now possible to get important insights into management practices and environmental effects affecting cattle productivity and product quality on a much larger scale – data like body weight at slaughter, carcass fat, age at slaughter, season, animal category, and much more. Even though analyzing big data can be challenging, it has multiple benefits and provides valuable information to make informed business decisions.
Data Collection in Cattle Production and Research
Advancements in animal sensors and the improved ability to monitor individual animals and their environments have provided many possibilities for collecting animal behavioral data. Individual animal records such as weights, treatments, and carcass characteristics, along with data regarding daily feed deliveries, diet ingredients, group weights, and movements, provide significant amounts of data useful for analysis.
There are also activity monitoring systems like accelerometers or real-time location systems to determine if the cattle movement is within an expected range. This helps in identifying patterns related to physiological changes or any disease. This enormous pool of data is valuable for predictive analysis.
Predictive Analysis Using Big Data
Predictive analysis using the collected information helps make specific decisions for the future. It involves data mining and machine learning to develop useful patterns and predict how cattle will perform in relation to health and growth. Certain predictive models are helpful in predicting things like the possibility of diseases, risk factors for disease, and failure to finish a production cycle due to sickness.
As part of predictive analytics, a target variable is chosen, data is managed and partitioned, algorithms are created and refined, and then classifiers are compared for accuracy. There are different classification algorithms used to predict outcomes – bayesian classification, random forests, logical regression, and decision trees are a few of them. A data analyst will best know the type of model to be used to predict a specific outcome.
Evert step in this process is equally important to provide accurate information and help make better decisions.
Defining Target Variable
The target variable is the outcome to be estimated or predicted, and it has to be carefully selected. The datatype used for the target variable will determine the predictive analytic model used. For example, ordinal data can be used to depict the yield grade or clinical illness score.
Once the target variable is chosen, the next step is to narrow the problem’s scope and identify the specific data needed and potential models to make decisions.
Data Partitioning
Data partitioning is a method to ensure that the new model being developed is accurate and can be used for new data sets. It involves partitioning the data into training, revising, and validation/testing data sets. The data is randomly segregated into datasets prior to model building, and the amount of data in each data set varies with the project goals. For example, if the data consists of various observations on individual cattle, then each dataset might contain randomly allocated cattle data.
Data Balancing
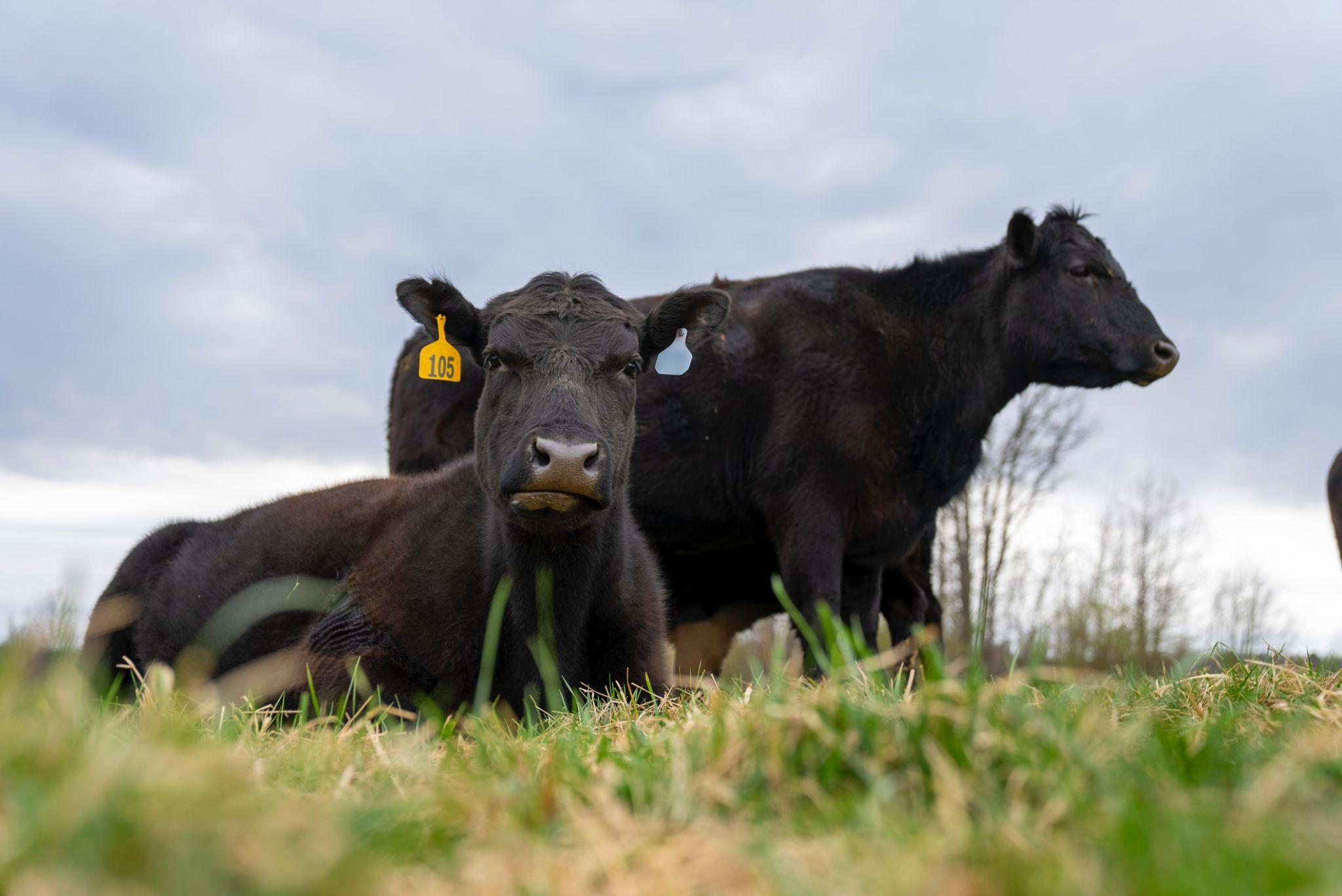
Two black cows resting in a field
Data balancing is one of the final considerations before building the model. It uses the frequency of specific outcomes to adjust the data set for more accuracy. Rare outcomes like mortality though not frequent have an immense economic impact, so modifications using data balancing can play an essential role before building the model.
The predictive analytic framework will help create a setting to evaluate many models and determine the best fit for a specific situation and a target variable. Through systematic use of these data, information can be generated to improve decision-making regarding livestock production, health, and welfare, thus increasing the genetic gain in the long term.
Data Analytics And Genomic Selection
The main aim of the beef industry is to improve the quality (marbling, tenderness, and flavor) and quantity(carcass weight) of the meat. Data analytics methodologies used in genomic selection, which are vital for predicting beef breeding values like backfat thickness, carcass weight, and marbling score, are highly beneficial in the beef production industry.
Genomic selection refers to the process of evaluating phenotypic data and genotypes of dense single nucleotide polymorphisms (SNPs) to estimate the effects of SNPs from a training population and subsequently to predict the genetic values of selection candidates based on their genotypes.
Reproduction efficiency is yet another highly profitable trait for the beef industry. Genomic selection can also help improve the predictive accuracy and genetic gain of fertility traits. The accuracy of genomic prediction increases the probability of a successful genomic selection.
There are several analytical approaches to predict the genetic values based on the data – these models mainly differ in the assumptions concerning the distributions of the effects of genetic markers. Ridge regression, genomic best linear unbiased prediction (gBLUP), and Bayesian shrinkage are a few selection models used. The performance of the statistical methods used depends on the traits analyzed.
Adopting genomic selection is more challenging in beef cattle than in other livestock animals due to the presence of multiple breeds and crosses and diverse environments. But, as a result of Big Data analytics, researchers and producers can understand where variability exists within operations, allowing them to make more informed decisions even in systems with considerable heterogeneity.
If you want to leverage the power of data analytics into your business, contact AgBoost. We are a data analytics company that provides easy-to-use tools to aggregate and analyze data and help make intelligent decisions. Contact us today to drive your business with data!